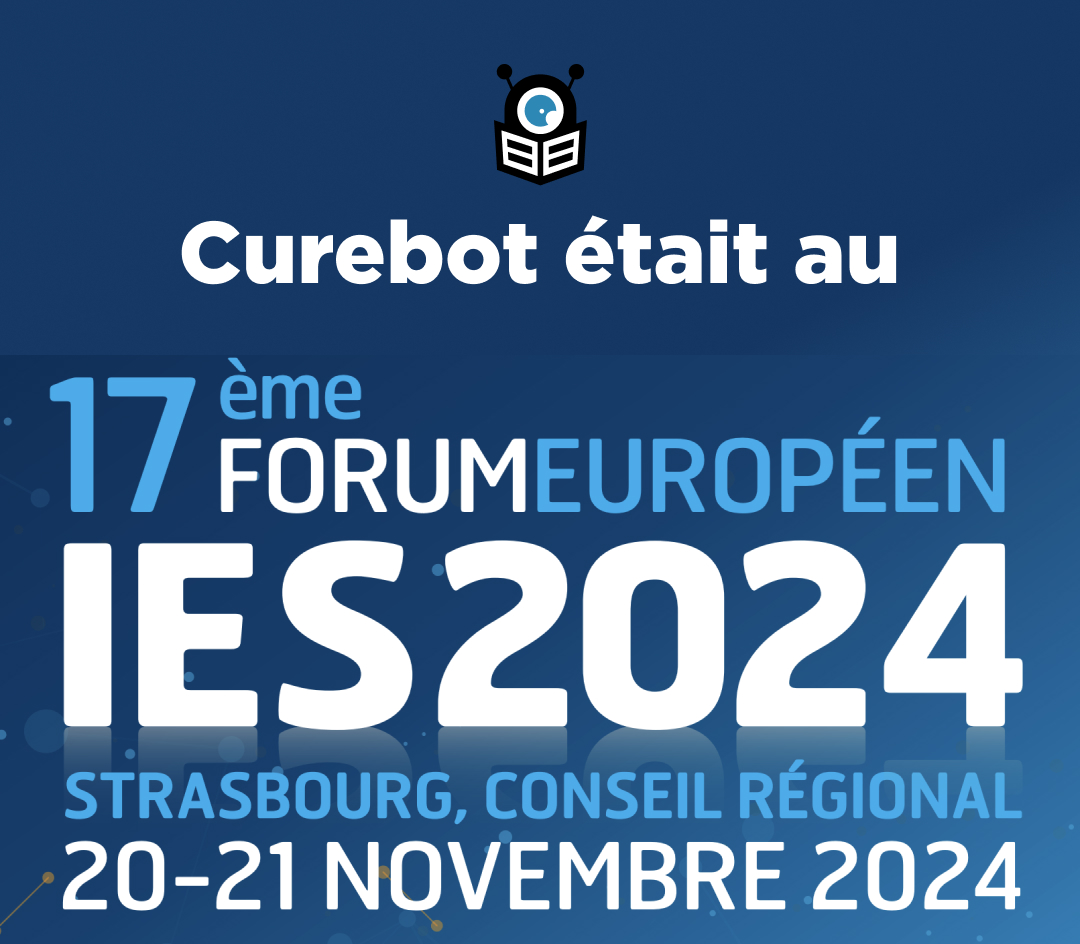
Back from the IES 2024 Forum: Business and Artificial Intelligence
A huge congratulations to Ophélie Garnier, to all the members of 3AF, and to the Grand Est Region for organizing this extraordinary event, which we can only regret is not held annually, given its rich contributions to the entire profession.
For my part, I had the pleasure of being able to participate alongside Jordan JeamBenoit, Caroline Martin of Onera, Pierre Memheld of the University of Strasbourg, in the restitution of the working group initiated by Franck Bourgine of the DGAC. This group was able to bring together academics, researchers, industrialists and software publishers to carry out an initial work of consolidation and analysis of the use cases of generative AIs in the information management cycle. Affaire à suivre !
Undoubtedly, generative AI will have been at the heart of many of the presentations at the IES 2024 forum.
Some lessons and thoughts following the forum:
There was a great similarity in the levels of experimentation, adoption, and industrialization of the use of generative AI within the organizations that took advantage of the IES forum to share their feedback. Many monitoring and/or business intelligence teams jumped on the generative AI bandwagon and launched experiments; jumped on the roller coaster of the generative AI hype cycle. They discovered with amazement, euphoria, and excitement the editorial capabilities of generative AI. However, they were also confronted with their limitations: errors, hallucinations, or failures of generative AI revealing the essential need to verify all productions presenting a level of criticality in the information management cycle. Some of these teams, few of them, are already at the 3rd stage of Gartner's hype cycle, consolidating their first productive use cases.
A consensus on the potential contributions of generative AIs in the different phases of the information management cycle:
Many use cases upstream of the synthesis phase:
-
-
- Sourcing assistance,
- Assistance with keyword detection,
- Assistance in building an ontology,
- Assistance with framing, expressing needs,
- Extraction of concepts or named entities,
- Qualification of the presence of themes in a list of given resources.
-
With acceptable levels of confidence and reliability of results but which continue to require the presence of an expert, an analyst to correct, refine, validate the outputs of the LLMs.
Results are still far too random in all phases of analysis and support for decision-making, action plan recommendations, communication or influence.
The strong organizational challenges associated with the development of the maturity of organizations in terms of the adoption and the capacity to derive tangible benefits from AI:
-
- Internal awareness program,
- Implementation of a Test & Learn program,
- Budget,
- Development of an internal technical environment aligned with data, security and IT issues associated with the integration of generative AI.
A low level of attention paid to issues of sovereignty, confidentiality, and the security of sensitive information and corporate secrets. This is all the more surprising considering the attention these issues received only ten years ago, particularly with the use of American search engines, the sharing of internal documents on the messaging platforms of these same players, and the justified issues and concerns of information security.
Whether through web interfaces or LLM providers' APIs (OpenAI, Gemini, Copliote, etc.), you reveal as much information about your interests through the prompts you run as through your search engine queries. The same goes for the documents you share for analysis and synthesis, which end up on servers at the very least. Worse still, these documents feed into LLMs and are integrated into LLM learning.
In my opinion, using the secure services of these players, "SecureChatGpt" and their ilk, with their promises of non-exploitation of resources and prompts, is not a satisfactory alternative for strategic monitoring and business intelligence. These promises (during the election period) are only binding on those who believe in them.
Thus, with a few rare exceptions, the shared feedback relied on the use of non-European AI, with data transferred outside of European territory and therefore without any real data security. Among the exceptions are the Orange group and its fortunate employees, who benefit from secure, self-hosted access to a set of LLM models to be able to experiment on a secure rooftop and with internal data; and two groups or research centers with a strong technical dimension.
For my part, a real concern raised at the end of the IES Forum concerns the future of the skills and knowledge of monitors, analysts and experts in the years to come: how will they be able to continue to develop their knowledge and strengthen their skills? While a consensus seems to have been established on the current limits of generative AI, particularly with regard to their analytical capabilities, another point is also unanimous: language models (LLMs) are proving to be extremely effective for producing summaries, syntheses, state of the art or even first levels of analysis. These tools can thus greatly facilitate the work of experts, analysts and monitors by saving them valuable time.
In our organizations, where the quest for productivity, savings, and performance is omnipresent, the integration of generative AI into everyday tools seems inevitable. Once these technologies have fully integrated the functional coverage of employees' tasks, it will only be a short step before managers expect their teams to produce summaries or deliverables in a fraction of the time previously allocated to them. For example, a state-of-the-art review of a technology, which previously required a scientific or technical expert to complete for several weeks, could now be generated in 30 minutes. In terms of pure production, the appeal is obvious, and the calculation of the time savings seems indisputable.
However, this pragmatic approach to productivity overlooks a fundamental element: the knowledge contributions for the human who creates this state of the art. Yann Le Cun did not become a world leader in machine learning by simply reading a state of the art generated by an AI. Reading resources, analyzing studies, pulling threads, exploring related work, and serendipously discovering new information – sometimes not immediately useful but valuable in another context – are essential steps for developing one's skills and enriching one's expertise.
It is by diving deep into studies, asking questions, and investigating that we build true mastery. Generative AI, however powerful it may be, cannot replace this human process of learning and intellectual exploration.
I invite you to discover the magnificent work Vu, lu, su by Jean-Michel Salaun: Simply being exposed to information (vu) is not enough to truly understand and assimilate it. It is necessary to take the time to read (lu) and analyze (su) to gain real knowledge. Reading is not just about passively consuming information, but about questioning it, cross-referencing it with other knowledge, and integrating it into personal reasoning.
This encourages the individual to become an actor in their own education and not just a recipient. Let us be clear: raw information is not the same as knowledge. Knowledge involves a process of selection, analysis, and reflection, which necessarily involves reading and in-depth study.
Finally, I share with you this article by Laura Hazard Owen, journalist at the Nieman Journalism Lab: “ I’m a journalist and I’m changing the way I read news. This is how. " which explains why she wants to return to a real reading of the articles: " For the past few years, I've read tweets about articles, but not the entire articles. I've read screenshots of those same articles that point out only the most scandalous details. Of course, extracting the best bits from an article makes for the best social media posts. But too often, I don't click. So I end up with the shocking little sentence from the article... and nothing else. Instead of trying to form my own opinion about the story told in the article, I just ingest other people's reactions. . "
And you, will you read the syntheses and summaries of LLMs or the complete studies and articles?
Follow us on Linkedin